The 2025 edition of the Virtual Winter School on Computational Chemistry is proudly sponsored by the School of Chemistry at the University of Edinburgh.
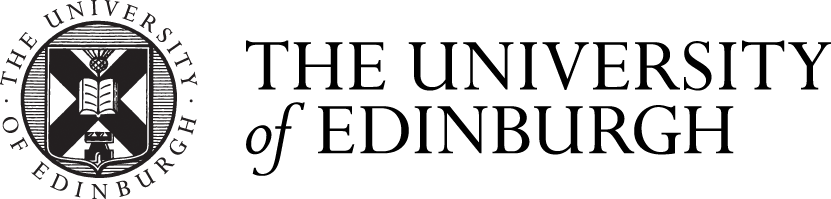
The Cooper Union for the Advancement of Science and Art is pleased to provide support for the 2024 VWSCC through a generous donation from Alan Fortier.
We thank Leibniz Institute for Catalysis (LIKAT) and CECAM for their support.